
Speculations
by Joanne McNeil
Turning Poetry into Art: Joanne McNeil on Large Language Models and the Poetry of Allison Parrish
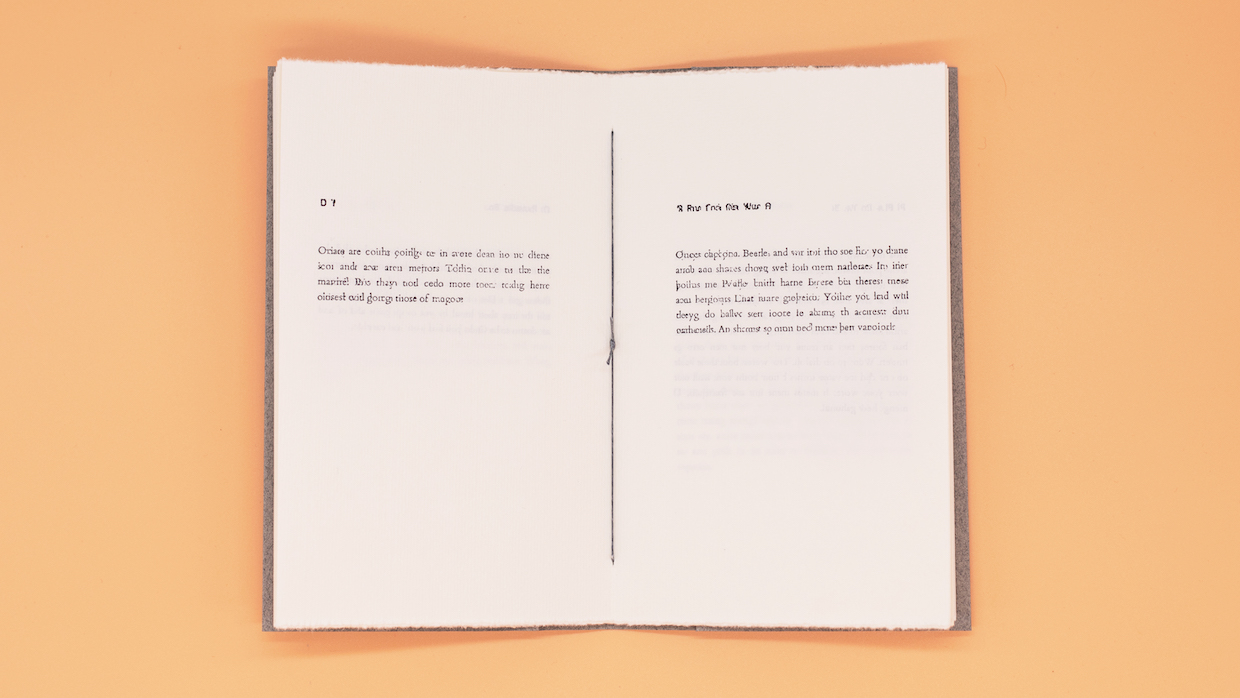
An application like ChatGPT is “taking the last 20 years of the internet and chewing it up, then producing a system that draws from that,” Allison Parrish explained when we spoke over Zoom last month. To the poet and programmer, generating content with large language model (LLM) neural nets is like “powering an engine with the methane that comes from decomposing corpses in a graveyard.”
Few artists working today have Parrish’s depth of experience with generative text. You have likely encountered her work online, especially if you were active on Twitter in the “Horse_ebooks” era. Early on in her career, she was featured in publications like The Village Voice and The Guardian for building bots like @everyword, which, from 2007 to 2014, tweeted “every word” in the English language in alphabetical order—or quite close to it. In recent years, Parrish has published acclaimed books of computational poetry, including Articulations (2018) and Wendit Tnce Inf (2022). She is currently developing a solar-powered device for generating poetry with a “radically small language model.” Her practice might fall under the heading of “AI art” (given the rubbery definition of what “artificial intelligence” even is), but no one would mistake what Parrish creates for Midjourney-made Wes Anderson-ified Dune trailers or any other turbo-pastiche novelties entered as a prompt and produced with the click of button.
I’ve been thinking a lot about Parrish’s work and that of other artists who have engaged with generative art long before OpenAI released ChatGPT to the public in November 2022 (which destined us to at least a year’s worth of thinkpieces on authenticity and the value of writing as thinking). The difference between what Parrish creates and the “AI” detritus swiftly clogging up the internet is obvious, but where is the line drawn?
Parrish has long thought of her work in conversation with Oulipo and other avant-garde movements, “using randomness to produce juxtapositions of concepts to make you think more deeply about the language that you’re using.” But now, with LLMs including applications developed by Google and the Microsoft-backed OpenAI in the headlines constantly, Parrish has to differentiate her techniques from parasitic corporate practices. “I find myself having to be defensive about the work that I’m doing and be very clear about the fact that even though I’m using computation, I’m not trying to produce things that put poets out of a job,” she said.
That risk, of course, isn’t fully hyperbole. In this year’s WGA strike, the union demands that its Minimum Basic Agreement with studios ensure that “AI can’t write or rewrite literary material; can’t be used as source material; and MBA-covered material can’t be used to train AI.” These boundaries, and similar demands from SAG-AFTRA, might inspire collective action from other organizations of writers, performers and creators. Professionals feeling the crunch range from audiobook narrators—swiftly being replaced with text-to-speech recordings—to literary translators, now regularly called in to copyedit shoddy Google Translate–generated drafts (labor that can be more of a lift than translating from scratch, but often for considerably less pay).
In a recent piece in The New Yorker, the author Ted Chiang likened potential uses of AI to
McKinsey, given how the management-consulting firm has helped “normalize the practice of mass layoffs as a way of increasing stock prices and executive compensation.” OpenAI and other LLMs offer corporations what Chiang calls an “escape from accountability.” AI, in this regard, doesn’t even have to work; it doesn’t matter whether there is any demand for generative outputs. From lie detectors to the Myers-Briggs personality test, corporations have an extensive history of adopting bullshit quantifications where it suits them. Just the same, LLMs might integrate swiftly into workflows and corporate decision-making already guided less by gut than by numbers—the television series that’s cancelled because ratings weren’t high enough, the book deal that doesn’t happen because of lackluster past sales.
But—again with “AI” being an annoyingly broad term—it is possible to experiment with these techniques locally without cost-cutting or profit-maximizing as the key objectives. Another artist I spoke with, who asked to be quoted anonymously, showed me samples of images he made with GANs (generative adversarial networks) trained on data sets that he gathered and cleaned. These images were “never mined from an external massive data set, and rather came from my own illustration and photography,” he told me. “It was more like seeing remixes of my own brain, which I do think has value as a daydreaming type of exercise.”
I’ve noticed that most coverage of the WGA strike zeroes in on the call to prohibit generative text displacing the labor of screenwriters. But just as crucial is the demand that existing work isn’t used as scrap metal to train these programs. It’s in the gathering of a corpus where LLM ethical violations are most glaring.
ChatGPT does not generate content from thin air. Training data serve as its ingredients, the flour and eggs to bake its cake. LLMs work by scanning a corpus for statistical relationships between words or elements in images; the generated output reveals a series of predictions it makes. (The training process, by the way, is astoundingly resource-intensive, with massive water and carbon footprints.)
OpenAI won’t say where its training data comes from, but it is obvious that social media is among its sources. The training data are wedding photos someone posted to Flicker in 2009, rants posted to Twitter about an airline delay in 2014, sexts and thirst traps and memes and the like by the billions from YouTube, TikTok and Instagram—the human receipts of lives lived on the internet. That’s why, beyond copyright, policy like the General Data Protection Regulation in Europe, which carves out data protection as a human right, could ideally serve as the basis for regulation. It’s not “fair use” when Facebook hoovers up our personal data and sells it, nor are targeted ads “transformative works.” Use of these data by OpenAI exacerbates existing data exploitation.
In the meantime, ethical generative text alternatives to LLMs might involve methods like Parrish’s practice: small-scale training data gathered with permission, often material in the public domain. “Just because something’s in the public domain doesn’t necessarily mean that it’s ethical to use it, but it’s a good starting point,” Parrish told me.
For Parrish, the ideal outcome of generative text is “that you produce something new, something that hasn’t been seen before, because these tools take you out of that conventional process of composition.” Take, for example, another of Parrish’s former bots, The Ephemerides, launched in 2015, which randomly selected an image from NASA’s OPUS database and posted it to Twitter along with a short, computer-generated poem. Two works available from Project Gutenberg, Astrology: How to Make and Read Your Own Horoscope by Sepharial and The Ocean And Its Wonders by R. M. Ballantyne, served as the training data.
If you were following the bot when it was live, amidst your Twitter feed you’d see an image from an outer space probe and a poem, suggesting the pensive inner monologue of sentient space craft:
That it sounds like an independent voice is the product of Parrish’s unique authorship: rules she set for the output, and her care and craft in selecting an appropriate corpus.
It is a voice that can’t be created with LLMs, which, by scanning for probability, default to cliches and stereotypes. “They’re inherently conservative,” Parrish said. “They encode the past, literally. That’s what they’re doing with these data sets.”