
Continue Watching
Exploring how and why we watch. by Jane Schoenbrun
What Does Netflix’s Algorithm Want?
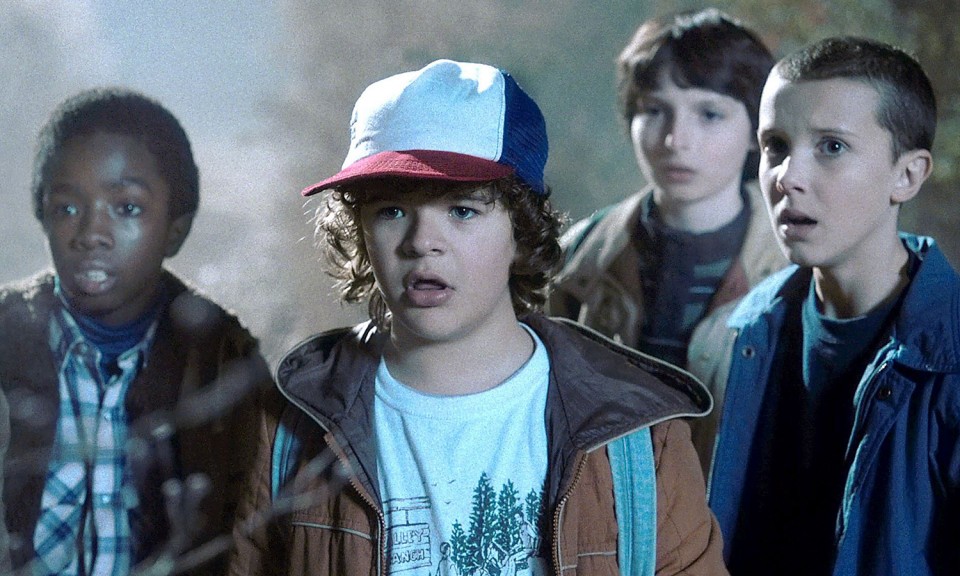
Back in June, Netflix’s VP of Product Innovation Carlos A. Gomez-Uribe and Chief Product Officer Neil Hunt co-published a paper entitled, “The Netflix Recommender System: Algorithms, Business Value, and Innovation.”
It’s a fascinating read, and if you care at all about the future of film as an artform, a fairly troubling one.
With their 19-page paper, Gomez-Uribe and Hunt provide a rare peek under the hood of Netflix’s inner-workings and technological developments, and go on to discuss the company’s business priorities and philosophy. The basic takeaway? Netflix has built an insanely complex and powerful recommendation system. This sucker has algorithms inside its algorithms.
I won’t spend too long overviewing that system, as a lot has already been written about how robust it is (I’d point you to this overview from The Atlantic, which calls the system peerless outside of Facebook’s Newsfeed algorithm).
Basically, the Netflix recommendation system works from a core concept of personalization. Back in 2007, when Netflix’s business was still DVDs in red envelopes, they employed a comparatively simple ratings-based recommendation system: an algorithm that predicted how much you’d like a new title by comparing your taste against others. But sometime after 2009, the company invested in a new, more complex and personalized infrastructure, in which films are broken down into over 75,000 hyper-specific sub-genres. Your individual preferences are inferred based on your watch history, and personalized recommendations are made from these all-encompassing sub-genres.
To create this system, Netflix hired dozens of freelancers to catalog every single movie in their catalog in a staggeringly complex database. These freelancers work from a massive 36-page training manual that guides them through the classification of nearly every aspect of every film. They rate how sexually suggestive the film’s content is, how gory, how conclusive or ambiguous the plot is, even the “moral status” of each lead character. This in turn inspires what you’re recommended.
So suffice to say, when Netflix tells you that you like “Dark Dramas with Strong Female Leads,” you better believe a lot of work went into arriving at that conclusion.
But in their paper, Gomez-Uribe and Hunt don’t just overview their system’s power. They dig into the question of why this algorithm is so important to Netflix’s business model. They share that the switch to personalization has not only resulted in users watching more stuff on Netflix, but an increase in customer satisfaction as well (as evidenced by a downturn in cancelled subscriptions). All in, they estimate that, “the combined effect of personalization and recommendations save us more than $1 billion per year.”
Later on, they share another impressive statistic: “Our recommender system… in total influences choice for about 80% of hours streamed at Netflix. The remaining 20% comes from search.”
Think about that for a moment.
80% of the stuff people are watching on Netflix is stuff that Gomez-Uribe and Hunt’s system is recommending. That means that the vast majority of the time, people don’t come to Netflix with a specific movie in mind. They’re not reading critics to decide what to watch, or browsing the BFI’s Top Movies List, or getting recommendations from friends. They’re logging onto Netflix and trusting that the system will present them with a suitable option.
Gomez-Uribe and Hunt argue that this is a net good for everyone: for Netflix’s business, it’s good to the tune of one billion dollars. For Netflix’s customer base, it’s good in that it provides an ideal user experience.
And they argue that it results in a greater overall diversity of consumption. Through this system, users are able to uncover and watch smaller niche titles specific to their interests that they might not become aware of otherwise. If you like boxing documentaries, you’ll see more boxing documentaries. If you like small indies about sad white guys who drink, you’ll see more small indies about sad white guys who drink.
What Gomez-Uribe and Hunt don’t get into is the reverse side of this coin: the cultural danger inherent in a feedback loop user experience. The stuff brought up in April Joyner’s recent essay for Marie Claire about Netflix’s troubling grouping of African American titles.
But from a business perspective at least, it’s hard to deny that the Netflix recommendation engine is kicking ass on an unprecedented scale. Sure, corporate curation is no new game, but I can’t imagine any system has ever come close to this level of influence. When you shopped at Borders or Tower Records back in the day, how often did you leave with stuff from their Recommended section? I’d wager it wasn’t 80% of the time.
The key difference here is that on Netflix, the recommendations are personal. This isn’t some lowest-common-denominator list passed down from headquarters. This isn’t the featured album at Starbucks or the new single that major label payola is cramming down your throat. It’s an entertainment cocktail prepared perfectly and specifically to your tastes, desires, and moral character.
What could be wrong with that?
Well if you’ve been reading this column regularly, you probably already know. It all comes back to that core tension at the heart of culture today: art vs. commerce.
Because it’s not a stretch to say that at this point Gomez-Uribe and Hunt — or perhaps more accurately, the machine that they’ve built — is the most influential curator of its generation. (Sorry, Hans Ulrich Obrist). But consider the goals of this robotic curator, and how those goals represent a complete break from the curatorial tradition.
This curator does not seek to provoke or challenge, nor to educate, nor to expand our understanding of art. It doesn’t seek to introduce audiences to daring work. It doesn’t hold a lens to the political and social issues of our time.
Instead, this curator’s only goals are those of commerce: to keep feeding customers more of what they want, and in turn, to help Netflix continue to grow and fortify its business.
In the conclusion section of their paper, Gomez-Uribe and Hunt get prosaic, arguing that their system serves not just a business purpose, but a moral one as well. I’ll quote them at length.
Humans are facing an increasing number of choices in every aspect of their lives — certainly around media such as videos, music, and books, other taste-based questions such as vacation rentals, restaurants, and so on, but more importantly, around areas such as health insurance plans and treatments and tests, job searches, education and learning, dating and finding life partners, and many other areas in which choice matters significantly. We are convinced that the field of recommender systems will continue to play a pivotal role in using the wealth of data now available to make these choices manageable, effectively guiding people to the truly best few options for them to be evaluated, resulting in better decisions.
Their word choice here is telling. They want to surface the “truly best few options.” They want to help people make “better decisions.”
But can art really be evaluated on such an empirical scale, as scientifically as we might evaluate health insurance options? Can a machine really serve us the “truly best few option?” Does such a thing as even exist in the realm of art?
Sure, the Netflix recommendation system can zero in on what we enjoy the most. And it can feed that back to us via staggeringly specific and well-researched recommendations. But let’s not pretend that this will result in us making so-called, “better decisions.” All it will result in is an increase in our consumption and an increase in Netflix’s profits. Not to mention a decrease in us being exposed to art that falls outside our comfort zones.
So let me amend some of the language used in that final sentence to make Gomez-Uribe and Hunt’s conclusion more precise. Instead of “guiding people to the truly best few options,” let’s say, “guiding people to the truly safest few options.”
And instead of “resulting in better decisions,” let’s just say, “resulting in you not cancelling your Netflix subscription.”